LangGraph: Revolutionizing AI Workflows with Graph-Based Orchestration
May 8, 2025
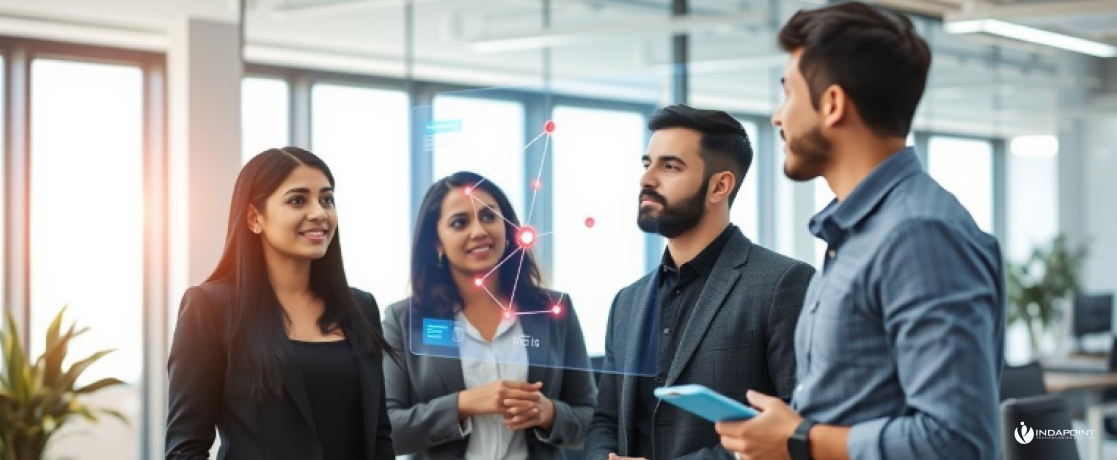
LangGraph transforms AI orchestration by introducing graph-based, stateful workflows that surpass the limitations of linear models like LangChain. With features like advanced state management, human-in-the-loop integration, and multi-agent support, it enables dynamic, adaptable applications. Ideal for complex use cases—such as research, customer service, and education—LangGraph empowers developers to build intelligent, scalable AI systems, despite its technical learning curve and setup complexity.
The Evolution and Foundation of LangGraph
LangGraph represents a significant advancement in AI orchestration frameworks by addressing the limitations of earlier tools such as LangChain. Traditional frameworks typically rely on linear execution models, while LangGraph introduces a graph-based approach, allowing for more dynamic AI systems. This framework enables developers to build stateful workflows that can maintain context, revisit previous decisions, and adapt to evolving scenarios.
Core Features of LangGraph
LangGraph boasts unique features critical for developing AI applications.
- Stateful Orchestration Maintains context across multiple interactions.
- Dynamic Graph-Based Workflows Supports cycles and branching decision paths, adapting workflows on-the-fly.
- Advanced State Management Features like state persistence and checkpointing enable sophisticated application states.
- Human-in-the-Loop Integration Facilitates human oversight at key decision points, enhancing AI decision-making.
- Multi-Agent Support Orchestrates complex interactions between multiple AI agents for collaborative tasks.
Practical Applications of LangGraph
LangGraph’s capabilities make it ideal for a variety of sophisticated applications.
- Research assistants utilizing iterative reasoning.
- Autonomous decision-making systems with multi-step evaluations.
- Complex customer service workflows featuring escalation paths.
- Content creation involving specialized agents.
- Educational tools adapting to user responses, contributing to personalized learning experiences.
Limitations and Considerations
While LangGraph offers powerful features, it also presents challenges.
- Technical Complexity A steeper learning curve and extensive configuration are needed for setup.
- Performance Considerations State management and checkpointing may impact application speed and resource use.
- Implementation Challenges Complexity arises during induction function usage and workflow visualization, potentially making integration with other tools more demanding.
When to Choose LangGraph
LangGraph is particularly beneficial under circumstances such as
- When applications need intricate workflows with conditional logic.
- In scenarios demanding robust state management across sessions.
- For projects requiring visual design for workflow maintainability.
- In cases where scalability exceeds simpler frameworks.
- When integration with monitoring tools like LangSmith is a priority.
Conclusion
LangGraph offers a transformative approach to building sophisticated AI workflows, making it an invaluable tool for developers aiming to create advanced, stateful AI applications. Designed to manage complex reasoning tasks and enable multi-agent collaboration, LangGraph is the perfect choice for those developing innovative solutions in AI, especially within the recruitment space.
Ready to elevate your AI workflows? 🚀 Whether you’re building smart assistants, dynamic content systems, or adaptive learning tools, LangGraph gives you the power to orchestrate complex, stateful processes like never before.