Two things that are crucial for successful web and mobile development projects are iterative methods that are used to create and execute projects and on the other side, the different ways of prototyping are used to quickly understand and reduce failures and misinterpretations.
With reliable and validated methodologies, IndaPoint responds to various market problems. To help you go forward, we recognize your organization’s needs and your requirements and then apply proven benchmarks and best practices.
Waterfall (Historically!)
When we started our journey almost fifteen years back, we were using the Waterfall methodology. It was working fine for us until the web development became an app development with sophisticated and advanced frameworks and technologies.
Software production itself was not easy back then either. Many development instruments needed advanced preparation, and the open-source or commercial software components, APIs, and online resources that exist today were not close anywhere. We had to build low-level items like opening connections to the database and multithreading our processing of data.
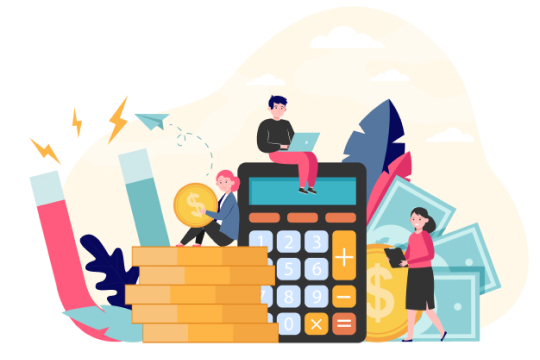
Teams were large for even simple applications, and communication instruments were small. What matched us was our technological requirements, and we leveraged them like the bible. When a standard changed, we will bring the company leaders through a rigorous evaluation process and sign off because it was costly to communicate improvements around the team and fix the code.
Scrum, Kanban, and other agile frameworks
Many agile frameworks provide specifics on development processes and agile development practices, aligned to a software development life cycle.
At IndaPoint Technologies, we prefer ‘scrum’ for our development cycles with a commitment to deliver a high-quality product with minimum supervisory and controlling leadership style.
We rely on a delivery pace known as a sprint and meeting frameworks, which contain the following aspects-
- Commitment, in which the team checks a list or backlog of user stories and calculates how much progress will be completed during the sprint.
- Regular stand-up calls, so that teams can exchange feedback on their status and plans for development)
- Planning where goals for sprints are established
Sprints conclude with a demo meeting where the device owner is shown the features, followed by a retrospective meeting where the team addresses what went well and what their method needs to be changed.
To support teams handle the scrum system, we also hire scrum masters or coaches.
Our Blogs: Feel the Beat of Innovation
Stay in sync with the latest in technology and business transformation.
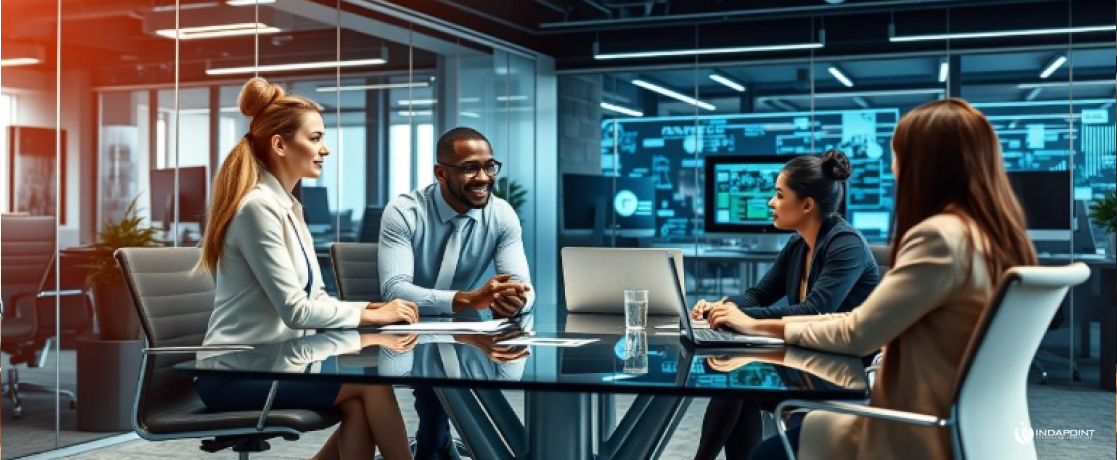
Unlocking the Power of LangChain: Integration Best Practices for Modern AI Applications
This blog explores how LangChain empowers developers to build advanced AI applications by bridging LLMs with real-world use cases. It outlines best practices like custom embeddings, robust error handling, modular design, and prompt optimization for seamless integration. With examples from enterprise assistants to customer support tools, it highlights LangChain’s impact and addresses key challenges like scalability and compliance—offering a roadmap for successful, future-ready AI development.
June 18,2025
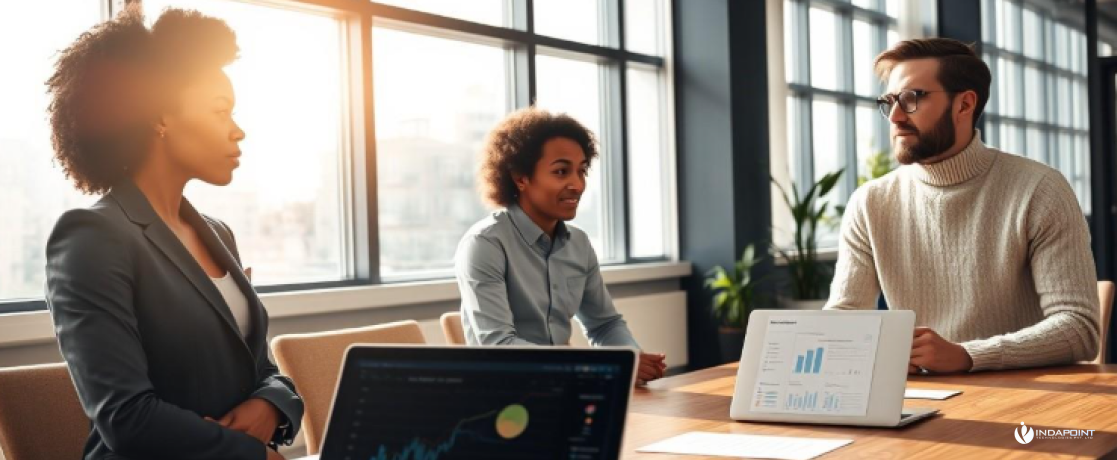
Claude AI Deployment Strategies: A Guide to Effective Integration and Optimization
This blog explores effective deployment strategies for Claude AI, Anthropic’s advanced, ethically aligned AI model. It outlines a phased approach—Preparation, Integration, and Optimization—for successful implementation. Real-world applications highlight Claude’s impact on DevOps, business operations, and developer productivity.
June 10,2025

Voice AI in 2025: Quietly Rewiring the Enterprise
Voice AI used to grab attention with flashy demos, talking avatars, and big promises. But in 2025, the focus has shifted to real, practical use. It’s no longer about showing off or testing ideas — it’s about getting real work done. Today, Voice AI helps in important areas like hospital support and customer service in different languages.
June 05,2025